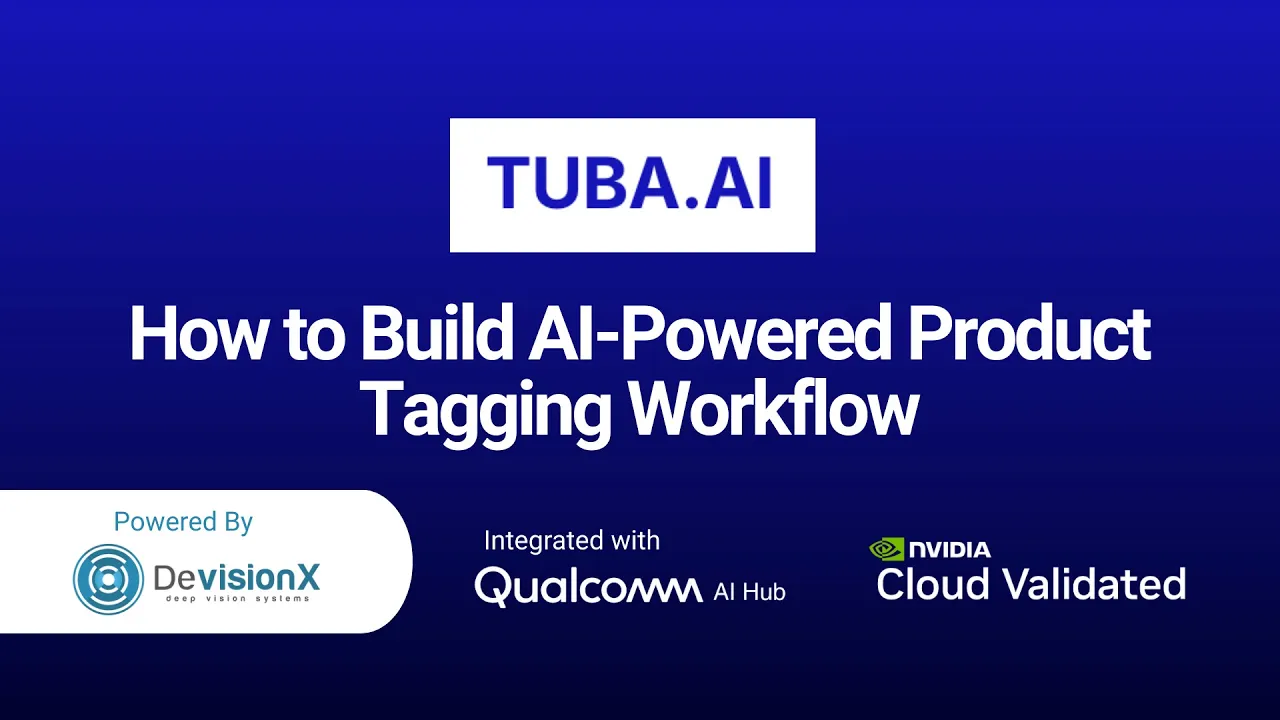
6 mins read
May 4, 2025
Industry Context: Scaling E-Commerce Efficiency with AI Vision-Language Models
To list a product on an e-commerce platform, it first needs to be described, not just with a title and price, but with structured, descriptive tags like color, material, style, and category. This process is known as product tagging, and it plays a crucial role in how products are organized, searched, and recommended across online stores.

For instance, Amazon’s marketplace boasts over 350 million products, with third-party sellers listing approximately 8,600 new items every minute, amounting to over 4.5 billion items annually. This deluge presents significant challenges:
Manual Tagging Bottlenecks: Manual tagging takes too long to keep up with fast-moving inventories
Delayed Time-to-Market: Slow onboarding processes hinder the rapid listing of new products, affecting sales opportunities.
Inconsistent Product Data: Varied data from multiple suppliers leads to discrepancies, impacting searchability and customer experience.
To stay competitive, e-commerce platforms and retailers must adopt AI-driven solutions that enable faster, more accurate, and scalable product onboarding.
The Opportunity: Automating Product Tagging with AI
Global players like Amazon are already adopting generative AI tools for product listings. A study of 2,500 SMEs in Europe using these tools showed:
77% reported significant time savings and increased efficiency.
74% noted improvements in content quality.
72% experienced increased profitability.
70% Boosted customer service.
69% expanded international market reach.
By integrating AI-powered Computer Vision and Vision-Language Models (VLMs) into tagging workflows, e-commerce platforms can:
Automatically generate consistent, high-quality tags
Enhance catalog structure, search, and recommendations
Eliminate repetitive manual tasks
Reduce costs and human error
Scale tagging workflows with zero bottlenecks
The Solution: Tuba.AI Workflow for Automated Product Tagging
Tuba.AI helps retailers and marketplaces accelerate and automate the product tagging process by turning product images into structured attribute tags using advanced vision-language models — all in a no-code, drag-and-drop interface.
To demonstrate how Tuba.AI can simplify and accelerate the product tagging process, we created a no-code, drag-and-drop workflow using real product images.
Key Steps Using Workflow on Tuba.AI
Image Upload:
We uploaded product images into the Tuba workflow for analysis.
Text Prompt Input:
We provided a customized prompt specifying what attributes or tags the model should extract from the images. This allows for customization based on industry-specific needs.
Make sure the prompt is understandable by the AI model. The prompt we used in the workflow is “Analyze the given product image and generate a list of relevant tags. Include attributes like color, material, style, category, and any notable features”.
Image-to-Text Processing:
After adding the image to the text API block, we used a state-of-the-art vision-language model like GPT-4o Mini, the system started to extract relevant product descriptions and attributes from the images according to the provided prompt.
Output Text Generation:
The processed data is structured into appropriate product tags, ready for integration into e-commerce platforms or inventory systems. Tuba’s drag-and-drop interface simplifies the setup, allowing businesses to deploy automated tagging without extensive AI expertise.
Results
We tested the workflow on a product image of a brown shirt. The model successfully extracted and returned structured tags such as:
Color: Brown
Style: Casual
Category: Shirt / Apparel
Features: Button-up, Long sleeves, Collar
The results came as shown in the image below.

The workflow can be customized to refine tag accuracy, ensuring that product attributes align with industry-specific standards.
The process was fast, consistent, and fully automated, eliminating the need for manual input while ensuring high-quality tag generation.
This confirms that Tuba.AI Workflow Builder can successfully extract accurate and descriptive text from any image to enhance the product tagging process.
Business Impact for E-Commerce Platforms & Retailers
Challenge | Traditional Approach | Tuba.AI Workflow Solution |
Tagging Time | Manual, slow, and error-prone | Automated tagging processing in minutes |
Consistency & Accuracy | Inconsistent across teams and products | Standardized AI-generated tags |
Scaling | Hard to manage as catalogs grow | Workflow adapts to high volumes and new products |
AI Expertise Gap | Requires a data science or development team | No-Code setup for catalog and operations teams |
Integration | Needs custom export tools | Integrated output ready for platforms |
Customization | Limited control | Custom prompts and tag structures per category |
Cost | High due to manual labor | Reduced costs through intelligent automation |
Why Choose Tuba.AI for E-Commerce Tagging?
AI Without the Overhead: Deploy vision-language models without hiring AI engineers.
Faster Time-to-Market: Get new products live faster with automated onboarding.
Customizable & Scalable: Tailor prompts per category and scale across thousands of SKUs.
Better Product Discovery: Improve SEO, in-platform search, and recommendation quality with better tagging.
Tuba.AI empowers e-commerce platforms to automate, scale, and standardize product tagging using advanced vision-language models saving time, reducing cost, and improving the customer experience.
Talk to our experts on how Tuba.AI can accelerate your catalog operations.
Want to see fast results? Try Tuba.AI Now!